Is Imitation All You Need? Generalized Decision-Making with Dual-Phase Training

We introduce DualMind, a generalist agent designed to tackle various decision-making tasks that addresses challenges posed by current methods, such as overfitting behaviors and dependence on task-specific fine-tuning. DualMind uses a novel “Dual-phase” training strategy that emulates how humans learn to act in the world. DualMind can handle tasks across domains, scenes, and embodiments using just a single set of model weights and can execute zero-shot prompting without requiring task-specific finetuning. DualMind demonstrate its superior generalizability compared to previous techniques, outperforming other generalist agents by over 50% and 70% on Habitat and MetaWorld, respectively.
SMART: SELF-SUPERVISED MULTI-TASK PRETRAINING WITH CONTROL TRANSFORMERS
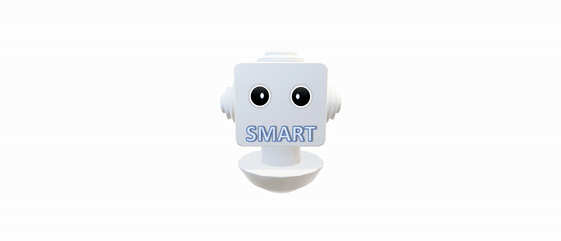
In this work, we formulate a general pretraining-finetuning pipeline for sequential decision making, under which we propose a generic pretraining framework Self-supervised Multi-task pretrAining with contRol Transformer (SMART). By systematically investigating pretraining regimes, we carefully design a Control Transformer (CT) coupled with a novel control-centric pretraining objective in a self-supervised manner.
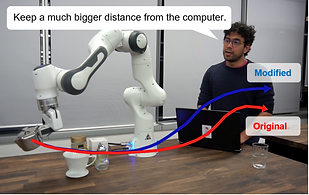
LATTE: LAnguage Trajectory TransformEr
​
This work proposes a flexible language-based framework that allows a user to modify generic robotic trajectories. Our method leverages pre-trained language models (BERT and CLIP) to encode the user’s intent and target objects directly from a free-form text input and scene images, fuses geometrical features generated by a transformer encoder network, and finally outputs trajectories using a transformer decoder, without the need of priors related to the task or robot information.
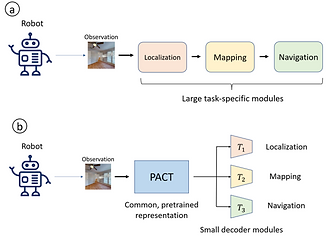
PACT: Perception-Action Causal Transformer for Autoregressive Robotics Pre-Training
​
Inspired by large pretrained language models, this work introduces a paradigm for pre-training a general purpose representation that can serve as a starting point for multiple tasks on a given robot. We present the Perception-Action Causal Transformer (PACT), a generative transformer-based architecture that aims to build representations directly from robot data in a self-supervised fashion. Through autoregressive prediction of states and actions over time, our model implicitly encodes dynamics and behaviors for a particular robot.
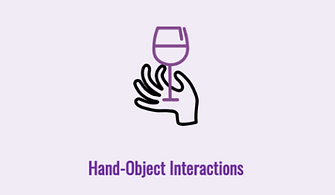
EgoMotion-COMPASS
2nd International Ego4D Workshop @ ECCV 2022
2nd Place: Object State Change Classification
2nd Place: PNR Temporal Localization
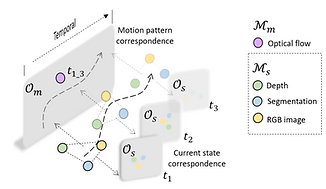
COMPASS: COntrastive Multimodal Pretraining for AutonomouS Systems
COMPASS aims to build general purpose representations for autonomous systems from multimodal observations. Given multimodal signals of spatial and temporal modalities M_s and M_m, respectively. COMPASS learns two factorized latent spaces, i.e., a motion pattern space O_m and a current state space O_s, using multimodal correspondence as the self-supervisory signal.

Reshaping Robot Trajectories Using Natural Language Commands: A Study of Multi-Modal Data Alignment Using Transformers
In this work, we provide a flexible language based interface for human-robot collaboration, which allows a user to reshape existing trajectories for an autonomous agent. We take advantage of recent advancements in the field of large language models (BERT and CLIP) to encode the user command, and then combine these features with trajectory information using multi-modal attention transformers.
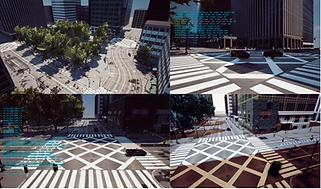
CausalCity: Complex Simulations with Agency for Causal Discovery and Reasoning
In this paper, we present a high-fidelity simulation environment that is designed for developing algorithms for causal discovery and counterfactual reasoning in the safety-critical context. A core component of our work is to introduce agency, such that it is simple to define and create complex scenarios using high-level definitions. The vehicles then operate with agency to complete these objectives, meaning low-level behaviors need only be controlled if necessary.
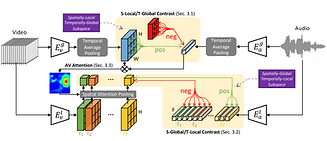
Contrastive Learning of Global-Local Video Representations
In this work, we propose to learn video representations that generalize to both the tasks which require global semantic information (e.g., classification) and the tasks that require local fine-grained spatio-temporal information (e.g., localization). We show that the two objectives mutually improve the generalizability of the learned global-local representations, significantly outperforming their disjointly learned counterparts.

LEARNING AUDIO-VIDEO REPRESENTATIONS BY CROSS-MODAL ACTIVE CONTRASTIVE CODING
In this work, we propose CrossModal Active Contrastive Coding that builds an actively sampled dictionary with diverse and informative samples, which improves the quality of negative samples and achieves substantially improved results on tasks where incomplete representations are a major challenge.
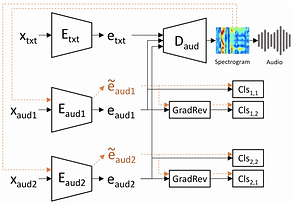
MULTI-REFERENCE NEURAL TTS STYLIZATION WITH ADVERSARIAL CYCLE CONSISTENCY
In this work, we propose an adversarial cycle consistency training scheme with paired and unpaired triplets to ensure the use of information from all style dimensions. We use this method to transfer emotion from a dataset containing four emotions to a dataset with only a single emotion.
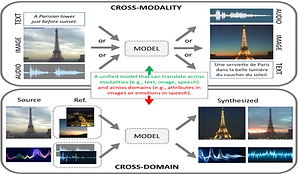
M3D-GAN: Multi-Modal Multi-Domain Translation with Universal Attention
​
We present a unified model, M3D-GAN, that can translate across a wide range of modalities (e.g., text, image, and speech) and domains (e.g., attributes in images or emotions in speech). We introduce a universal attention module that is jointly trained with the whole network and learns to encode a large range of domain information into a highly structured latent space. We use this to control synthesis in novel ways, such as producing diverse realistic pictures from a sketch or varying the emotion of synthesized speech. We evaluate our approach on extensive benchmark tasks, including imageto-image, text-to-image, image captioning, text-to-speech, speech recognition, and machine translation. Our results show state-of-the-art performance on some of the tasks.
Neural TTS Stylization with Adversarial and Collaborative Games
​
In this work, we introduce an end-to-end TTS stylization model that offers enhanced content-style disentanglement ability and controllability. Given a text and a reference audio as input, our model can generate human fidelity speech that satisfies the desired style conditions.
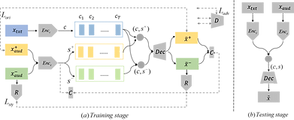


​Unpaired Image-to-Speech Synthesis with Multimodal Information Bottleneck
​
In this work, we introduce the problem of translating instances from one modality to another without paired data. Specifically, we perform image-to-speech synthesis for demonstration.
Click to play audio

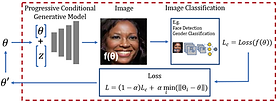
Characterizing Bias in Classifiers using Generative Models
In this work, we incorporate an efficient search procedure to identify failure cases and then show how this approach can be used to identify biases in commercial facial classification systems.
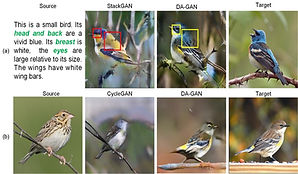
DA-GAN: Instance-level Image Translation by Deep Attention Generative Adversarial Networks
​
In this work, we propose a novel framework for instance-level image translation by Deep Attention GAN (DA-GAN). Such a design enables DA-GAN to decompose the task of translating samples from two sets into translating instances in a highly-structured latent space.
Pose Maker: A Pose Recommendation System for Person in the Landscape Photographing
​
In this work, we proposed pose recommendation system. Given a user-provided clothing color and gender, this system shall not only offer some suitable poses, but also assist users to take high visual quality photos by generating the visual effect of person in the landscape pictures.